Part 5: Is AI really that simple?
Updated on 12 December 2024
This post is part of the AI Apprenticeship series:
- Part 1: AI Apprenticeship 2024 @ DiploFoundation
- Part 2: Getting introduced to the invisible apprentice – AI
- Part 2.5: AI reinforcement learning vs human governance
- Part 3: Crafting AI – Building chatbots
- Part 4: Demystifying AI 
- Part 5: Is AI really that simple?
- Part 6: What string theory reveals about AI chat models
- Part 7: ‘Interpretability: From human language to DroidSpeak’
- Part 8: ‘Maths doesn’t hallucinate: Harnessing AI for governance and diplomacy’
By Dr Anita Lamprecht, supported by DiploAI and Gemini
Is AI simple?
‘Is AI really that simple?’ was the guiding question of week 5 of Diplo’s AI Apprenticeship online course, and it struck me. I had just begun writing this blog post by focusing my research on the meaning of the term ‘cognitive’, as in ‘cognitive proximity’, and its relation to knowledge, bias, frames, and schema – all terms used in previous lectures. When I looked up ‘cognition’, one of the first results was ‘schema (human cognition)’. Britannica defines ‘schema’ as a mental structure that guides our cognitive processes and behaviour.
Each schema is unique and depends on an individual’s experiences and cognitive processes. A schema acts as both a frame and a filter, shaping how we perceive and interact with the world around us, from categorising objects to making decisions and predicting outcomes. Schemas allow us to fill gaps and see the whole picture. Our semantic understanding plays an important role in this mental process.
Since we rely on existing knowledge to interpret new information, this highlights the profound impact of our cultural background and individual experiences on our perception of the world. In summary, our complex understanding (intellectual, emotional, biological, etc.) of relationships governs our behaviour. Similarly, in the field of AI, retrieval-augmented generation (RAG) systems utilise a form of schema by accessing and incorporating external knowledge into their output. Why did this strike me?
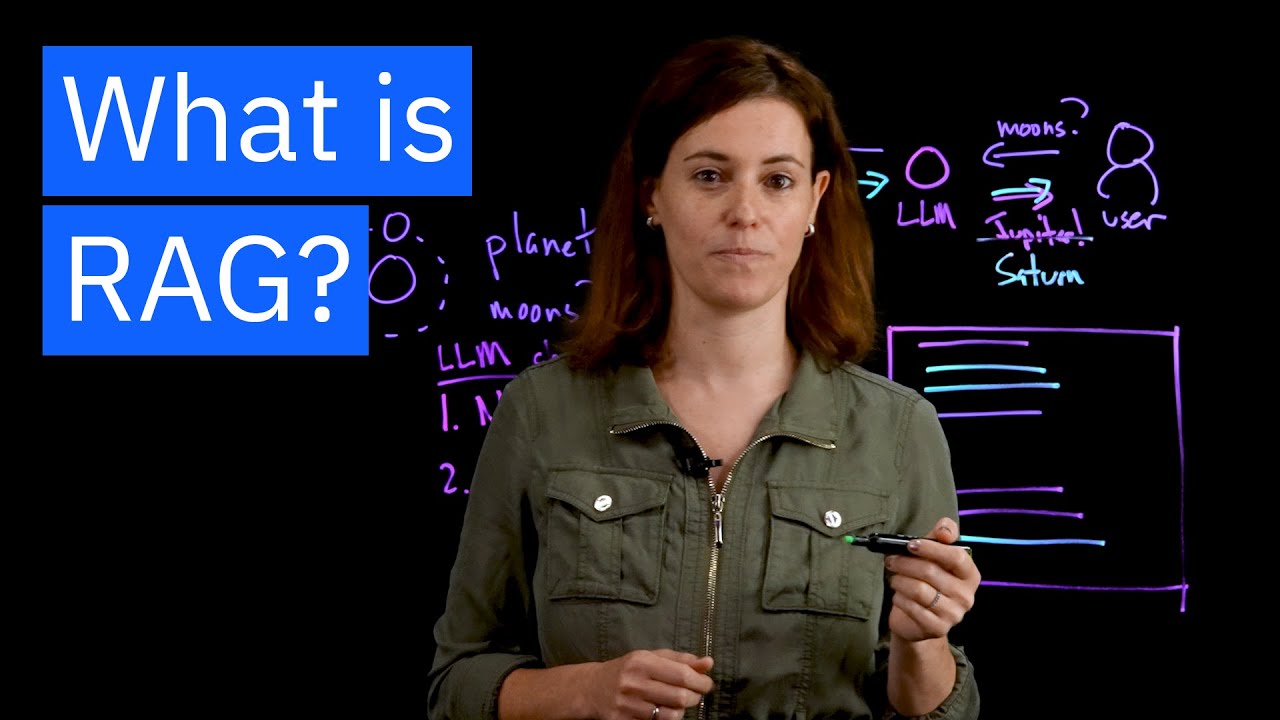
Is AI just a ‘Schema F’?
It struck me because there is an idiom in my German mother tongue called ‘Schema F’. This idiom stands for a formulaic, standardised approach, implying a rigid pattern or set of rules often followed without considering individual nuances or specific contexts. While schemas can streamline processes and provide mental shortcuts, they can also mask underlying complexities of a problem or situation, creating a false sense of simplicity. When I further researched the translation of ‘Schema F’, one translation was astonishingly, ‘is it that simple?’.
Both simple and complex
In the AI Apprenticeship course, we learned that, to some extent, the simplicity of AI lies in its accessibility. Within only one month of apprenticeship, we all had our own Diplo chatbots up and running. However, comprehending the mechanisms (schemas) of how our knowledge is processed is a much more complex task.
Neither humans nor machines process information and knowledge in a vacuum. Humans are influenced by emotions, social contexts, and individual experiences. Bias is a part of schema; like our knowledge, it is part of our humanity. AI primarily relies on syntax and probability, recognising patterns, analysing statistical relationships, and generating output based on learned rules.
While AI can mimic human language and even generate creative text formats, it lacks the deep understanding of meaning and context that characterises human thought. So, is AI really a ‘Schema F’, and is it really that simple?
Shifting weights
Perhaps the simplicity of access to this artificial knowledge-processing tool is what increases the complexity of human cognition. AI creates a feedback loop from its mathematical vector space into our human semantic space, subtly altering the weights we assign to different ways of knowing.
We begin to prioritise efficiency and data-driven insights, potentially overshadowing human intuition, emotional intelligence, and the nuanced understanding that comes from lived experience. This shift in weights can reshape our values, influence decision-making, and ultimately redefine what it means to be intelligent in an age of AI.
Eloquent uncertainty
In the course, we also learned that our admiration for eloquence has very deep implications. As AI chatbots exhibit high levels of eloquence without semantic understanding, our minds can easily be misled by a false impression of meaning, which lacks actual depth.
‘To confuse’ means to throw one’s mind into a state of uncertainty. Uncertainty is defined as the feeling or attitude that one does not know the truth, truthfulness, or trustworthiness of someone or something. When humans fall for AI’s eloquence, does it mean that we no longer trust in our own knowledge and abilities? Does AI influence our feelings about ourselves?
The smallest unit of analysis
To answer this question, we would need to delve into psychoanalysis, which is not my area of expertise. So, let’s shift the focus back to my perspective as a lawyer, which is governance. The smallest unit of analysis in governance is the individual. What is the smallest unit for AI?
The OpenAI natural language models don’t operate on words or characters as units of text, but instead use something in-between: tokens. Tokens are specific text segments that form common words, parts of words, or punctuation marks.
In the course, we tested OpenAI’s Tokenizer to better understand this mechanism. Each token has a unique TokenID, which allows models to process text as numbers. For example, ‘ai’ has the tokenID [1361], whereas ‘human’ has the tokenID [51527]. I conducted research to gain insights into GPT-4o’s vocabulary.
tokenID | Value | tokenID | Value | tokenID | Value | tokenID | Value |
0 | ! | 8 | ) | 27 | < | 65 | b |
1 | “ | 9 | * | 28 | = | 994 | 11 |
2 | # | 10 | + | 29 | > | 8780 | 111 |
3 | $ | 12 | – | 31 | @ | 18596 | § |
4 | % | 13 | . | 32 | A | ||
5 | & | 14 | / | 33 | B | 1361 | ai |
6 | ‘ | 15 | 0 | 64 | a | 51527 | human |
7 | ( | 16 | 1 | 65 | b |
Gemini’s key findings on GPT-4.o
I used Gemini to help reveal the structure and pattern behind my findings and their relevance for governance. (Please remember that a system like Gemini can make mistakes.)
According to Gemini, the vocabulary seems to prioritise punctuation marks, with ‘!’ taking the coveted spot of tokenID [0]. This suggests that the training data might be rich in expressive language, potentially from social media platforms like Discord. The model appears to have a strong affinity for code-related symbols and mathematical operators, hinting at possible inclusion of code repositories or technical discussions in the training data.
Letters seem to be organised alphabetically, with uppercase letters preceding their lowercase counterparts. This suggests a sensitivity to case and a potential emphasis on lexical processing. While numbers initially appear sequentially, the later placement of larger numbers like ‘11’ and ‘111’ suggests a more complex tokenisation scheme that considers frequency and possibly sub-word units.
Implications
These findings raise important questions about the model’s potential biases and strengths. Was it heavily trained on social media data, leading to a bias towards informal language or emotionally charged expressions? Does its exposure to code make it particularly adept at handling technical tasks or generating code snippets? Given the prevalence of exclamation marks, informal language, and code-related symbols, Discord emerges as a strong contender for a social media platform that may have significantly influenced this language model’s vocabulary.
While these findings offer a glimpse into the model’s inner workings, they also underscore the importance of transparency and critical analysis in AI.
Understanding even a model’s smallest units of analysis is crucial for identifying potential biases, interpreting outputs, and ensuring responsible use. They are part of the schema influencing our cognition, decision-making, and predictions of the world around us.
Sounds simply complex.
The AI Apprenticeship online course is part of the Diplo AI Campus programme.
Related blogs
Related events
Related resources
Subscribe to Diplo's Blog
The latest from Diplo and GIP
Tailor your subscription to your interests, from updates on the dynamic world of digital diplomacy to the latest trends in AI.
Subscribe to more Diplo and Geneva Internet Platform newsletters!
Diplo: Effective and inclusive diplomacy
Diplo is a non-profit foundation established by the governments of Malta and Switzerland. Diplo works to increase the role of small and developing states, and to improve global governance and international policy development.
Leave a Reply
Want to join the discussion?Feel free to contribute!