Which predictive method is better: Simple or sophisticated?
Updated on 23 August 2024
I’ve argued this many times: Prediction is dicey. Does close study and sophisticated methodology pay off compared to ‘back of the envelope’ predicting? In Everything is Obvious: Once You Know the Answer, Duncan J. Watts reports on studies he has carried out, comparing various kinds of predictive models, and testing them against basic heuristics – rules of thumb.
Take the outcomes of NFL football games. The ‘basic’ predictors were that (a) the home team statistically has some (measurable) edge, and (b) recent win-loss records provide information about the overall ‘health’ of a team. These two predictors were tested against two ‘betting markets’ and two ‘opinion polls’. What was the result?
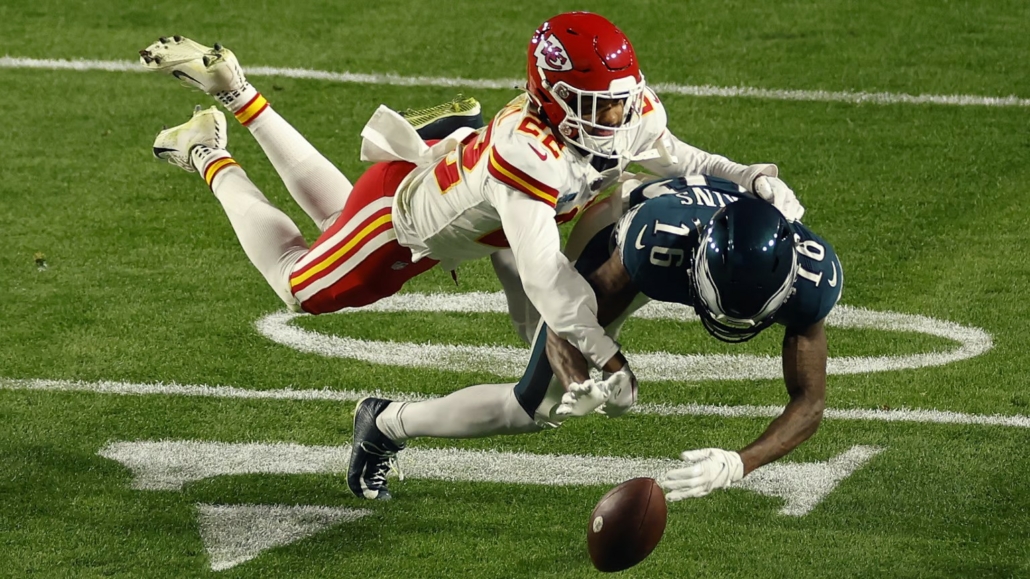
Here are the conclusions: ‘Given how different these methods were, what we found was surprising: all of them performed about the same.’ The best performing method was only about three percenntage points more accurate than the worst-performing method. Then they moved to baseball: ‘the outcomes of baseball games are even closer to random events than football games.
So they conclude: ‘There are strict limits to how accurately we can predict what will happen. […] it seems that one can get pretty close to the limit of what is possible with relatively simple methods. […] Predictions about complex systems are highly subject to the law of diminishing returns: the first pieces of information help a lot, but very quickly you exhaust whatever potential for improvement exists.’
In hindsight, this does not surprise me. The human brain is a rather imperfect machine for observing and predicting natural events. Yet, we have prospered by drawing simple lessons from experience. Nothing sophisticated, just ‘good enough’ to give us an evolutionary advantage.
Policy used to be made ‘on the back of an envelope’ by people who had a lot of practical experience. It was messy; it included a lot of ‘silent’ knowledge – hunches, intuitions, lore. They were replaced by ‘modellers’ who had a lot of experience in statistical methods.
I shall pass lightly on the fact that mathematical manipulations necessarily destroy information as they elaborate it (see On the Accuracy of Economic Observations by Oskar Morgenstern). The fact remains that ‘making more realistic models’ tends to disaggregate a limited number of basic variables, in an attempt to deepen the understanding. Refinement of a limited number of independent variables trumps, when we might be better advised to be more inclusive of variables – even at the cost of consistency. Refinement is based on the unstated assumption that the past contains all that is necessary to know to understand the future, i.e. that nothing important will change. Instead, things may have structurally changed, and the time series we use no longer reflect the evolution.
What lessons are to be drawn? The first is: always check the performance of your predictors. We tend to remember our ‘hits’ and forget how many times we missed. The second would be to focus on what has changed foremost, rather than refine the understanding of the past as laid out in a given predicting model, thus expecting the past to replicate itself in the future. Re-engineering the model may be more productive than refining it. Of course, the new version may be a ‘jury-rigged’ affair, but that’s what life is about.
Related blogs
Related events
Related resources
Subscribe to Diplo's Blog
The latest from Diplo and GIP
Tailor your subscription to your interests, from updates on the dynamic world of digital diplomacy to the latest trends in AI.
Subscribe to more Diplo and Geneva Internet Platform newsletters!
Diplo: Effective and inclusive diplomacy
Diplo is a non-profit foundation established by the governments of Malta and Switzerland. Diplo works to increase the role of small and developing states, and to improve global governance and international policy development.
Leave a Reply
Want to join the discussion?Feel free to contribute!